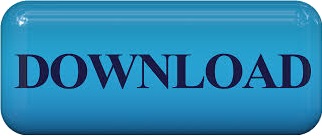

A wide range of numbers is necessary for neural network training for the weights, activations (forward pass), and gradients (backpropagation). Due to the limited number of bits used, it cannot store numbers with infinite precision thus, there will always be a trade-off between range and precision. However, the binary system cannot represent some values accurately. The floating-point format is the standard way to represent real numbers on a computer. I’ll stick to the basics that help frame the difference between the floating-point data types. Anatomy of a Floating-Point Data TypeĪ fantastic 11-minute video on YouTube describes how floating-point works very well.
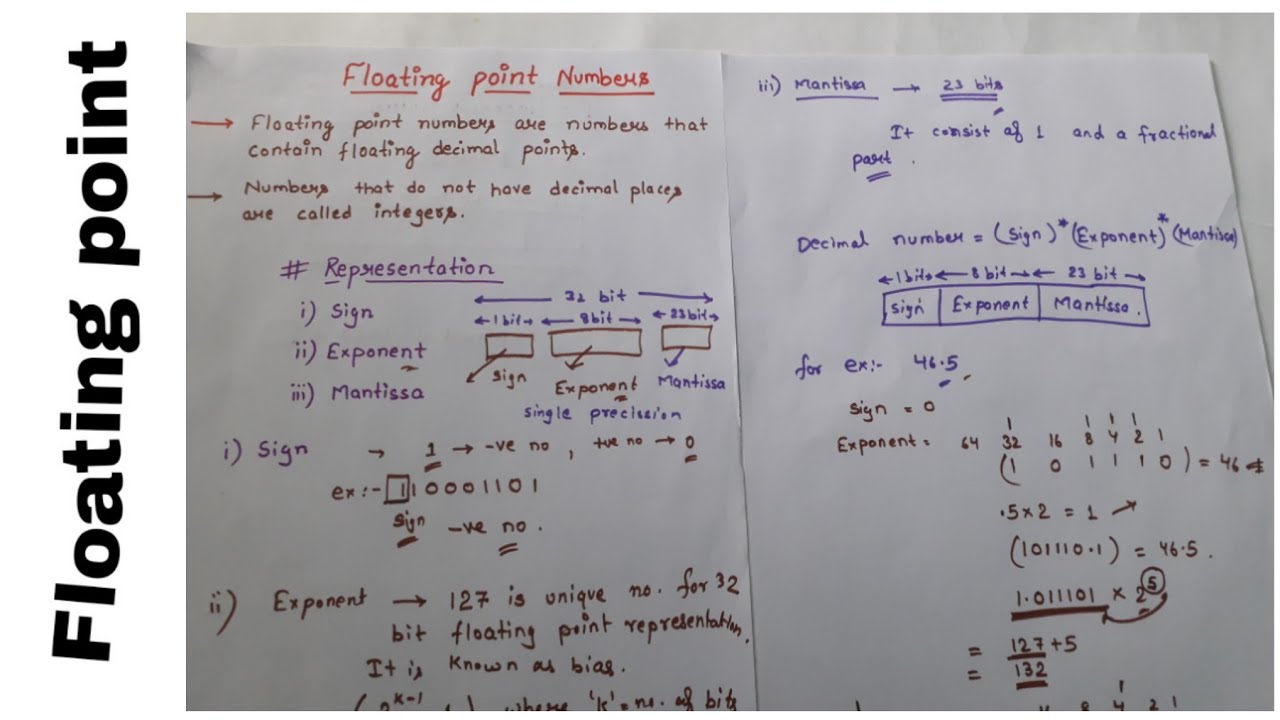
What do these numbers mean? We have to look at the anatomy of the different floating-point data types to understand the performance benefit of each one better. Sparsity functionality support allows the A100 to obtain these performance numbers, and sparsity is a topic saved for a future article. Some floating-point data types have values listed with an asterisk.

Integer data types are another helpful data type to optimize inference workloads, and this topic is covered later. This overview shows six floating-point data types and one integer data type (INT8). I’m aware that NVIDIA announced the Hopper architecture, but as they are not out in the wild, let’s stick with what we can use in our systems today. Let’s look at a spec sheet of a modern data center GPU. However, modern CPUs and GPUs support various floating-point data types, which can significantly impact memory consumption or arithmetic bandwidth requirements, leading to a smaller footprint for inference (production placement) and reduced training time. By default, neural network architectures use the single-precision floating-point data type for numerical representation. Most machine learning is linear algebra at its core therefore, training and inference rely heavily on the arithmetic capabilities of the platform. Part 4 focused on the memory consumption of a CNN and revealed that neural networks require parameter data (weights) and input data (activations) to generate the computations.
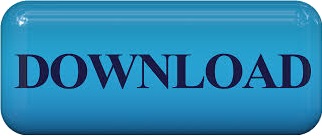